Digital Twin Ontology: Unlocking Efficiency and Innovation
In the dynamic world of industry, two concepts stand out: Digital Twin and Ontology. In this article, we dive into their significance, evolution, and impact. Whether you’re a seasoned professional or a curious learner, this read promises fresh perspectives on industrial efficiency.
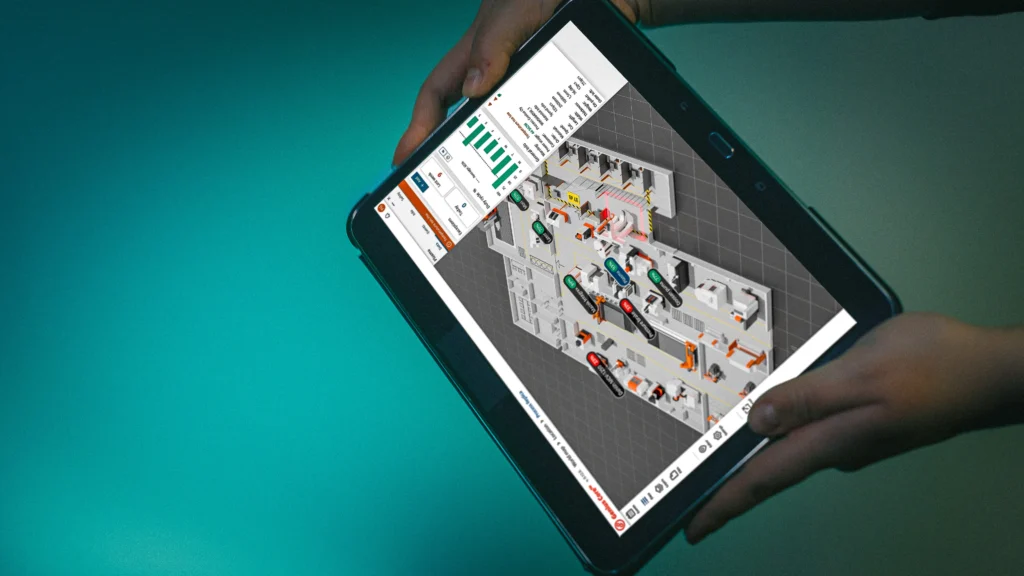
What is a Digital Twin ontology?
In the field of information science and knowledge representation, an “ontology” refers to a formal and explicit specification of concepts, entities, and relationships within a specific domain of interest. Essentially, it outlines the structure and semantics of a knowledge domain by organizing concepts into a hierarchy and defining their properties and interconnections.
Central to the concept of digital twins, ontology, along with other techniques and technologies, constructs digital twin models. As businesses and leaders increasingly embrace digital twin technologies, there has been a rapid surge in adoption. This trend aims to leverage new opportunities for competitive advantage and effectively navigate the vast amounts of generated data.
The term ‘Digital Twin Ontology’ refers to a structured framework or system that defines and organizes the concepts, relationships, and properties related to Digital Twins [1]. Essentially, it serves as a standardized vocabulary or a set of rules that aids in understanding, modeling, and sharing information about Digital Twins across various applications, industries, and contexts. This ontology plays a crucial role in facilitating communication, interoperability, and collaboration among stakeholders involved in Digital Twin development, deployment, and usage.
By incorporating ontology within Digital Twin systems, data can be organized in a coherent and consistent manner, making it easier to access, retrieve, and analyze. Ontology enables semantic interoperability by establishing common vocabularies and shared meanings, allowing different systems and components to accurately exchange and interpret data—even if they were developed independently or originate from diverse sources.
From concept to reality: Understanding the evolution of Digital Twin ontology
To understand the direction of “Digital Twin Ontology” development we need to have a brief look into Evolution of Digital Twin with key milestones [2]:
- Early conceptualization (2000s): It involved creating virtual representations of physical assets to simulate their behavior, monitor performance, and optimize maintenance.
- NASA’s use in space missions (2002): NASA pioneered the use of Digital Twin technology in space missions with its Remote Exploration and Experimentation (REX) program.
- Adoption in industrial IoT (2010s): In the 2010s, Digital Twins gained traction in industrial sectors such as manufacturing, energy, and transportation, driven by the rise of the Internet of Things (IoT).
- Siemens’ innovation (2015): Siemens introduced its vision of the Digital Twin concept, emphasizing the integration of virtual and physical worlds to enable predictive maintenance and optimize product performance.
- Advancements in Data Analytics (2016-present): Recent years have seen rapid advancements in data analytics techniques, including machine learning and artificial intelligence.
- Expansion into new industries (2018-present): Digital Twin technology has expanded beyond traditional industrial sectors into areas such as healthcare, smart cities, and infrastructure.
- Standardization efforts (2020s): Standardization bodies and industry consortia have begun developing frameworks and guidelines for Digital Twin implementation to ensure interoperability, security, and scalability.
Taking all of the above into account and taking into account the needs of the main actors in the process of industrial digitalisation, we could reduce “Digital Twin ontology” to a way of organising and structuring data in a way that delivers meaning. That meaning helps to derive new insights which can be used to realise tangible value from data.
We at Process Genius believe that the key direction for the development of Digital Twin Ontology within general concepts such as Industry 4.0 and Industry 5.0 will be centred around these three parameters “interoperability, security, and scalability”.
Overcome data noise and craft your own ontology
When you create a complete Digital Twin that replicates a specific domain with data from multiple systems (e.g., manufacturing site, pulp mill, power plant), you’re essentially crafting your own ontology.
Here we have to mention mainly the taxonomies and classifications used in the Digital Twin ontology [3]:
- Functional taxonomy or multi-functional platform. This taxonomy categorizes Digital Twin components based on their functionality or purpose within the system. Example categories may include data acquisition, data processing, simulation, analytics, visualization, and control.
- Domain-specific taxonomy or universal connectivity functionality. Digital Twins are applied across diverse domains such as manufacturing, healthcare, transportation, and smart cities. For example, in manufacturing, domain-specific taxonomies may include terms related to production processes, equipment types, and quality metrics
- Application-specific taxonomy or lay out functionality. Within each domain, Digital Twins may serve specific applications or use cases, each requiring its own taxonomy. For example, in manufacturing, application-specific taxonomies may include terms related to predictive maintenance, production optimization, supply chain management, and product lifecycle management.
- Hierarchical Taxonomy or role-based functionality. Taxonomies can be hierarchical, with broader categories at the top level and more specific subcategories beneath them. For example, a hierarchical taxonomy for a manufacturing Digital Twin may start with broad categories like “Asset Management,” “Process Optimization,” and “Quality Control,” with subcategories such as “Equipment Health Monitoring” and “Production Scheduling” beneath them.
Our years of experience in 3D Digital Twin development has allowed us to create a platform, which allows us and our customers to practically apply Digital Twin Ontology and evaluate its benefits on concrete real-world use cases.
It’s worth noting that Digital Twin implementations that use ontologies to improve decision making, efficiency and innovation can raise questions and cause some confusion. If you’re struggling to find answers, we’re happy to help!
Sources:
- [1] Microsoft. (2023). What is an ontology? Retrieved from https://learn.microsoft.com/en-us/azure/digital-twins/concepts-ontologies (read 3 May 2024).
- [2] Verdict Media Limited. (2021). Digital Twins – Timeline. Retrieved from https://www.verdict.co.uk/digital-twins-timeline (read 3 May 2024).
- [3] van der Valk, H., Haße, H., Möller, F., Arbter, M., Henning, J., Otto, B. (2020). A Taxonomy of Digital Twins.
Head of R&D
For most of his career, Janne has been involved in developing solutions for the digitalisation of industry. In his work, he has grown from a problem solver of IT issues at a startup to a customer-oriented manager. Currently, he serves as a senior manager for Process Genius and also holds roles as a project manager for research projects and research coordinator.